UNM researchers use artificial intelligence to improve electrical grids
A research team led by UNM Professor Manel Martínez-Ramón of the Department of Electrical and Computer Engineering is developing an algorithm that will help electrical grids use energy more efficiently. The team includes Graduate Research Assistants Nestor Pereira and Miguel Hombrados Herrera as well as UNM Professor Andrea Mammoli and Associate Professor at the Universidad Carlos III de Madrid Vanessa Gómez Verdejo.
The project is a part of the New Mexico EPSCoR Smart Grid Center grant, funded by the National Science Foundation. According the EPSCoR website, “The current National Science Foundation (NSF) EPSCoR Track 1 project in New Mexico establishes a novel, interdisciplinary research center—the NM SMART Grid Center—which is pursuing next-generation electric power production and delivery through creation of a SMART electric grid (one that is Sustainable, Modular, Adaptive, Resilient, and Transactive).”
Electrical grids can control how energy is created, stored, and distributed. The smart grid that the EPSCoR project is developing will use artificial intelligence to automatically adjust energy storage based on how much energy will likely be needed under specific conditions. Weather is a very important factor in these decisions; as Pereira writes, “There are several articles that show that temperature has a strong correlation with energy consumption since the utilities that consume most of the energy in the house are temperature regulators.”
To help smart grids predict energy needs accurately, Martínez-Ramón’s team is devising an algorithm that will tell a smart grid what weather and power load data to base its decisions off of on any given day. Martínez-Ramón explains, “The motivation of selecting data for machine learning is to produce a training that is valid for the next day, but not necessarily for the day past tomorrow or the next month. What we do is to choose training data which is more similar to the data used for tomorrow’s forecast, train a machine, produce a test, and then we repeat the process [the] next day with new data. This improves the performance of the machines because we do not use data that does not convey information about what is going to happen tomorrow. Plus, the resulting learning machines are more compact, since the volume of training data is reduced.” By making the smart grid’s predictions more accurate, the algorithm allows energy to be used more efficiently.
To design the algorithm, Martínez-Ramón’s research team has been working with large quantities of weather and aggregated power load data provided by ISO New England, a non-profit organization that oversees electricity markets in New England. Resources from the Center for Advanced Research Computing are being used to process this data. Pereira reports, “Predictions require big historical datasets and have to run about 160 scripts for different areas in the New England area and different prediction methods to check our success with our data selection algorithm. CARC computer power and [the] number of nodes available really helps!”
Pereira hopes that this technology may be used to support the increased use of renewable energy sources. He comments, “I’m a big supporter of renewable energies! But they lack on reliability. If there is no sun one day, we don’t generate solar energy. If the wind is missing, we can’t use our windmills. With a smart grid, energy can be stored in batteries and, based on weather forecasts, this lack of energy production on days as such can be substituted by energy stored in batteries, allowing our grid to be completely independent from the national electrical grid.”
The algorithm is expected to be published by the end of the summer of 2020. The New Mexico EPSCoR Smart Grid Center is a five-year project that began in September of 2018. As a team lead in the Decision-Support division of EPSCoR, Martínez-Ramón will continue designing programs that help smart grids make better decisions.
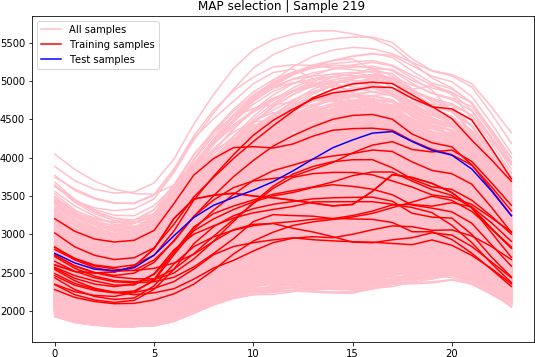